Application of GMM-UBM with an embedded AANN in the acoustic emission signal recognition
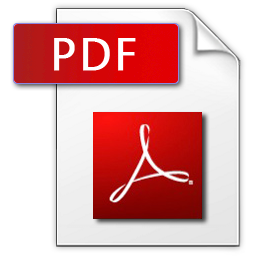
Cheng Xinmin1, Li Jing2, Jiang Yunliang2, Gao Ge2
1School of Information Engineering, Huzhou Teachers’ College, Huzhou 313000, Zhejiang, PR China
2School of Information Science and Engineering, Southeast University, Nanjing 210096, Jiangsu, PR China
In this paper, we propose to recognize the Acoustic Emission (AE) signal, by using a Gaussian Mixture Model-Universal Background Model (GMM-UBW) with an embedded AANN. The AANN based GMM-UBW combines the learning ability of neural network and strong distribution capabilities of GMM. And, it trains the model parameters alternatively in order to approach the maximum likelihood. For illustrating the effectiveness of the proposed recognition method for the AE signal, an experiment is conducted. In the experiment, three cases of AE signal are considered, namely with no rub impact, slight rub impact and serious rub impact. The experimental results reveal that the AANN based GMM-UBW outperforms the GMM, with respect to the recognition rate, for any case of AE signal. For the case of slight rub impact, the GMM-UBW and that with embedded AANN both have the worst recognition performance, among the three cases. And, the proposed method has the biggest improvement for this case.