Face recognition based on the invariant single training sample
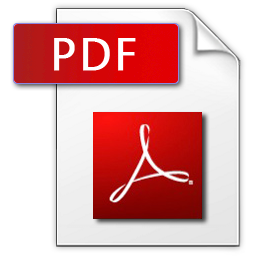
Zhaonan Yang1,2, Shu Zhang2
COMPUTER MODELLING & NEW TECHNOLOGIES 2014 18(12B) 70-78
1College of Computer Science and Technology, Harbin Engineering University No.145, Str. Nantong, Harbin, China
2College of Computer Science and Technology, Heilongjing University of Science and Technology No.l2468 Str. Puyuan, Harbin, China
Despite the constantly change of human face pose, illumination, expression, and occultation, one major problem of the face recognition technique arises from the difficulties of gaining training samples. When everyone just can gain an image for face recognition, the training samples are so insufficient that the extracted feature vectors can not support the whole face sample subspace and the performance drop is expected. This problem is called the face recognition with the single training sample and has received significant attention during the past years. Researchers have proposed image-strengthen method, sample-expansion method, and generic learning framework, etc, which mostly aim to expand the number of the training samples by using computer techniques to create several combining virtual images based on the original one. Therefore, the problem simply becomes a general face recognition problem. However, these methods result in enlarging the calculation volume and requiring bigger storage space. It also needs to be retrained once a new person is put into system. These problems make it extremely difficult to popularize these methods. In this paper, we try to exclude training and to extract features directly from the hybrid Taylor-ATMT, which has constructed a set of invariants. The recognition errors caused by the change of human face expression, illumination and partial occultation could be reduced after projecting it to wavelet space to lower the dimension, and then classify categories with the use of Bayesian Decision Theory, which results in a better effect. Experiments are implemented on YALE and ORL face databases to demonstrate the efficiency of the proposed approach. The experimental results show that the average recognition accuracy rates of our proposed method which are higher than those of previous methods.