Multi-view object recognition based on sparse representation
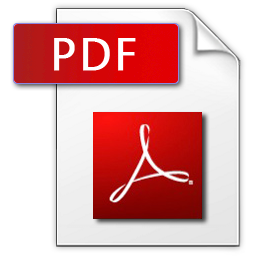
Jin-jin Cai, Bo Liu, Wei Yao
COMPUTER MODELLING & NEW TECHNOLOGIES 2014 18(12B) 163-168
College of computer science and technology, Agriculture University of Hebei, Baoding, China
In recent years, sparse representation has emerged as a powerful data representative model to draw much attention. In term of the intrinsic structured characteristic of signal itself, this model decomposes a signal as a linear combination of a few atoms from an over-completed dictionary. As it turns out, we obtain the parsimonious representation of signal with regularization by different sparsity-inducing norms. Through the adaptivity and robustness of sparse representation, it is well applied to the field of signal and image processing. In this paper, the problem of recognizing an object from its multiview images with unconstrained poses and context was considered. A novel framework called metasample-based supervised dictionary learning for multi-view object recognition exploiting the sparse property of intrinsic information was proposed. Experimental results demonstrate that the proposed algorithm exhibits better performance than the recent state-of-the-art methods.