Study on prediction and spatial variation of PM2.5 pollution by using improved BP artificial neural network model of computer technology and GIS
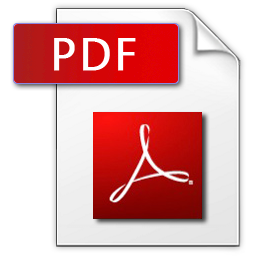
Ping Zhang1, Tao Zhang2, Liang He3, Cailiang Wei1, Sui Luo1, Huan Miao1, Peishu Huo4
COMPUTER MODELLING & NEW TECHNOLOGIES 2014 18(12C) 107-115
1School of Environmental and Chemical Engineering, Xi`an Polytechnic University, Xi`an 710048, China
2Institute of Grassland Science, Northeast Normal University, Key Laboratory of Vegetation Science, Ministry of Education, Changchun 130024, China
3Xi'an Environmental Monitoring Station, Xi`an 710054, China
4School of Environment, Tsinghua University, Beijing 100084, China
Atmospheric pollutant PM2.5 seriously harm to human health, to accurately predict its pollution condition, can avoid or reduce the risk of pollution events. In this study, we used the different algorithms and number of hidden layer neurons to improve BP artificial neural network model of computer technology, coupling GIS to evaluate the impact of different algorithms on the prediction and spatial variation of PM2.5, the results showed that, mean relative error and correlation coefficient of monitoring and predictive value by the six different algorithms and three different number of hidden layer neurons, were 14.02% and 0.97, respectively, indicating that improved BP artificial neural network model can be used to predict PM2.5 pollution. Optimization algorithm of trainrp and trainlm had the highest prediction accuracy while the number of neurons in the hidden layer is 20. In contrast, the same algorithm, different number of hidden layer neurons had a greater influence on the simulation of PM2.5. Spatial variation of PM2.5 by different algorithms and Inverse Distance Weighted interpolation method has various degrees of difference from that of the observed, although the simulation of north-central high risk area and southeast low risk region are basically consistent to interpolation analysis of monitoring data.