ARTIFICIAL NEURAL NETWORK MODEL OF FORECASTING RELATIVE HUMIDITY IN DIFFERENT HUMID AND ARID AREAS OF CHINA
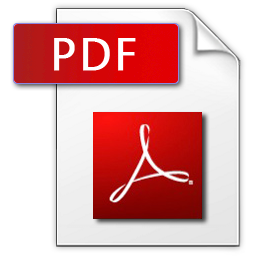
Zhenfang He1, 2
1Cold and Arid Regions Environmental and Engineering Research Institute, Chinese Academy of Sciences, Lanzhou, China, 730000
2University of Chinese Academy of Sciences, Beijing, China, 100049
The objective of the present study is to build different models forecasting the daily mean relative humidity (MRH) values in China with the help of the meteorological parameters. A back-propagation artificial neural network (BPANN) models was employed to identify the relationship between meteorological factors and the relative humidity in China. Weather data 1-day lag was the input layer variables, including (1) the highest atmospheric pressure, (2) the lowest atmospheric pressure, (3) the average atmospheric pressure, (4) the average temperature, (5) the highest temperature, (6) the lowest temperature, (7) precipitation, (8) the average wind speed, (9) the maximum wind speed (the average wind speed over 10 minutes), (10) the utmost wind speed, (11) hours of sunlight, (12) the relative humidity. Experimental results: in the validation period for 1-day lead, the comparison of the prediction performance efficiency of the BPANN models indicated that the BPANN models with trainbr algorithm was superior to the remaining two ones (trainlm and traingdx) in forecasting the relative humidity time series in term of correlation coefficient (R). During the training and testing periods for 1-day lead, the best performance for the given problem was arid area, followed by semi-arid area, semi-humid area, and humid area respectively. The possible cause for the results was that the impact of these factors on the relative humidity in arid area was the largest, followed by semi-arid area, semi-humid area, and humid area, respectively. From the prediction results of MRHextrema, humid area was the first; semi-arid area was the second; semi-humid area was the third; and arid area was the fourth. From the prediction results of MRHextrema, trainbr algorithm was the best in arid area, semi-humid area, and humid area; but trainlm was the best in semi-arid area. So trainbr algorithm was further employed to predict MRH for 2, 3 or 4-day lead at Urumqi City. From the training and testing effects, 1-day lead was the best, followed by 2, 3 or 4-day lead respectively. In the prediction results of MRHextrema, the best was 2-day lead; the second was 3-day lead; the third was 1-day lead; and the fourth was 4-day lead. The BPANN model results will assist researchers determining meteorological parameters to forecast MRH.